Efficient Estimation of Propagator Anisotropy and Non-Gaussianity with MiSFIT
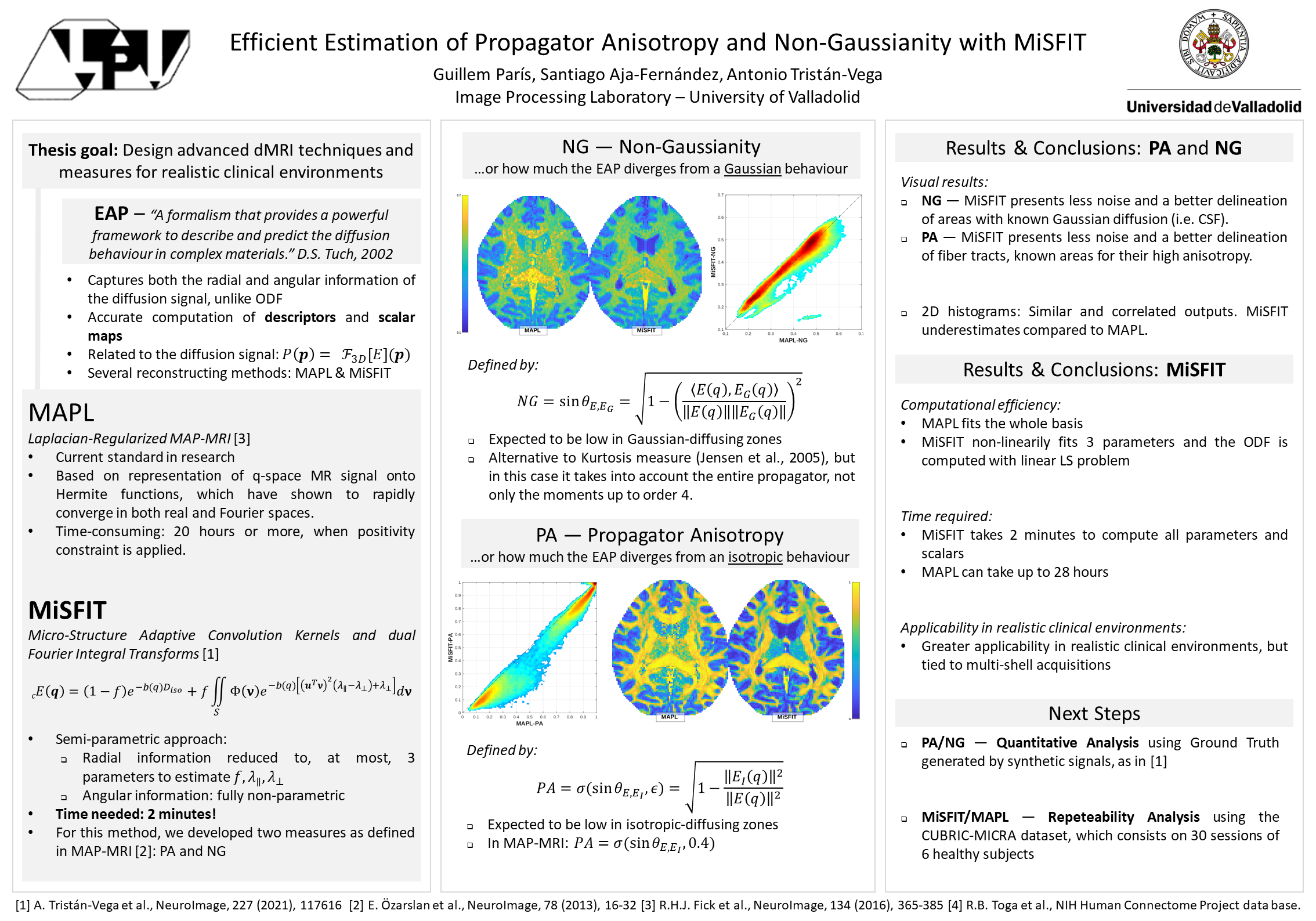
Poster presented at the I Annual Meeting of the Iberian Chapter of the ISMRM (2021)
Abstract:
The reconstruction of the Ensemble Average Propagator (EAP) from multi-shell samplings (MSS), one of the main challenges the diffusion MRI community is facing, enables an accurate computation of descriptors and scalar maps, critical for diagnosis, early treatment, and prediction of pathologic conditions. The novel semi-parametric MiSFIT [1] (Micro-Structure adaptive convolution kernels and dual Fourier Integral Transforms) has obtained certainly promising results in terms of accuracy, reliability, and computational efficiency. It is based on representing the EAP as a convolution of a fODF with an adaptive Gaussian kernel estimated from the diffusion signals averaged at each acquired shell.
Here we re-formulate, for the MiSFIT approach, two diffusion scalar indices previously introduced in [2], namely: Propagator Anisotropy (PA) and Non-Gaussianity (NG), which are defined as the EAP's deviation from an isotropic or a Gaussian one, respectively. Their importance resides in their potential to reveal micro-structural configurations. Trusting the good results obtained in [1] for other indices like the Return-to-Axis/Origin/Plane Probability, we expect PA and NG, as computed with MiSFIT, to improve the accuracy and reliability of the de facto standard in the state of the art, i.e. the Laplacian-Regularized MAP-MRI (MAPL) [3].
Up to now, visual assessments of the results indicate similar outcomes from both reconstruction models, as well as an increased accuracy in some case studies. However, it should be noted that, while MAPL needs up to 28 hours to fit its model, MiSFIT does it in less than 3 minutes.
This processing time reduction, strongly dependant on the computational efficiency, together with the MiSFIT's robustness to the MSS scheme, and a plausible improvement in micro-structure characterization, could mean the difference that definitely qualifies diffusion MRI to be incorporated into clinical settings.
[1] A. Tristán-Vega et al., NeuroImage, 227 (2021), 117616
[2] E. Özarslan et al., NeuroImage, 78 (2013), 16-32
[3] R.H.J. Fick et al., NeuroImage, 134 (2016), 365-385
[4] R.B. Toga et al., NIH Human Connectome Project data base. URL http://www.humanconnectomeproject.org