Noise estimation and diffusion signal reconstruction: From cradle to parallel imaging (MICCAI TUTORIAL 2010)
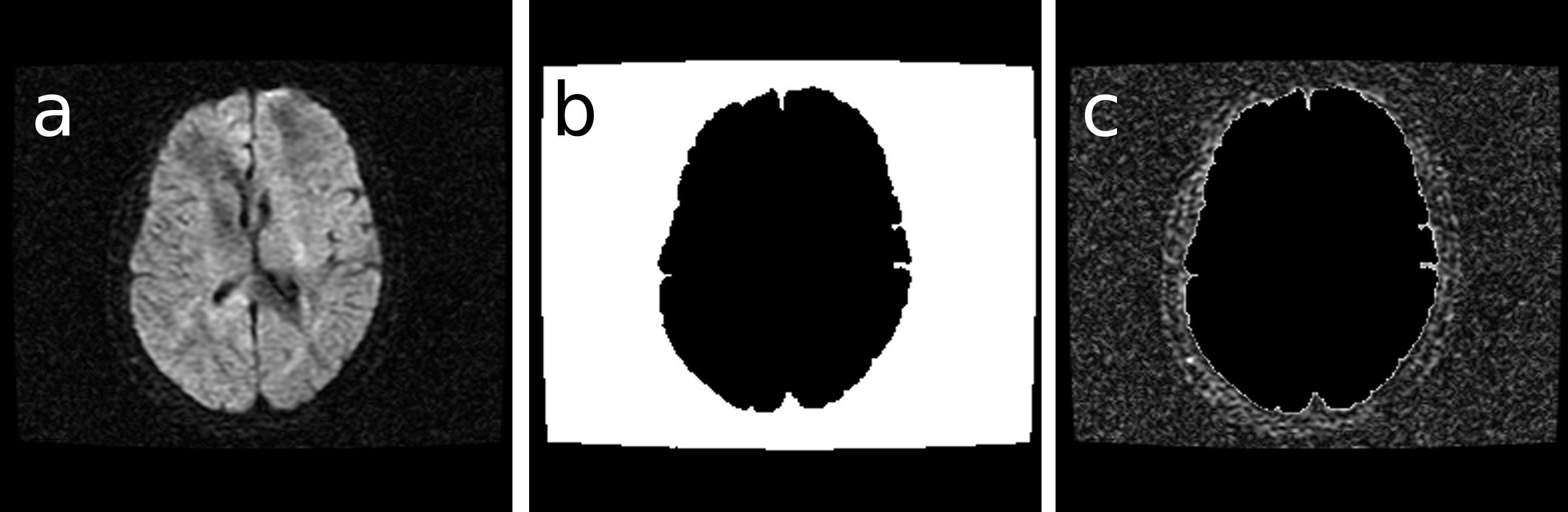
Slides from tutorial presentation in MICCAI 2010: Noise estimation and diffusion signal reconstruction: From cradle to parallel imaging. What type of noise infects the data and by filtering it out are we (black) magically creating something new?
Abstract: Noise is known to be one of the main sources of quality deterioration in magnetic resonance (MR) data. Not only visual inspection but also processing techniques such as segmentation, registration or tensor estimation in diffusion tensor MRI (DT-MRI) will be affected or biased due to the presence of noise. In High Angular Resolution Diffusion Imaging (HARDI) for instance, to achieve a speedup in the acquisition time the temporal averaging is reduced; as a consequence, the strength of the noise is increased proportionally to the square root of the speedup. Noise in the k-space in MR data is usually assumed to be a zero-mean uncorrelated complex Gaussian process in each scanner coil, with equal variance in both the real and imaginary parts. As a result, sometimes it is easy to derive the distribution of the magnitude data (as in single coil systems) and other not-so easy, as in multiple coil parallel reconstruction.
Many filtering methods have been proposed either to remove the noise or to estimate the signal out of the noise. Different approaches may be found in the literature: (1) general filtering methods (anisotropic diffusion filtering, total variation methods) and (2) methods based on the statistical properties of the signal and noise (LMMSE, ML...). The talk will concentrate on discussing the nature of noise and means to address the challenges associated with it.